How the brain learns to learn
/Artificial intelligence (AI) has rocketed ahead in its ability to recognize faces, drive cars, and make medical diagnoses. A remaining limitation is that AI requires hours or days of training to learn how to respond appropriately in each new situation. Humans, by contrast, can quickly "get" the crux of problems despite little direct experience. For a demonstration of your ability to do this, simply press play below:
Even if you've never played this game before, you were probably able to score pretty well on your first try. If you noticed the scoreboard, you likely sought out a strategy that would rack up points and prevent you from losing lives. This is an example of knowing how to learn in a new context by transferring over abstract rules from prior experiences. Humans instinctively "learn how to learn" in this way beginning in childhood.
Through deep learning, AI has exceeded human performance in the game above, called Breakout, and developed gaming strategies with an impressive level of sophistication.1 For example, AI has learned to win Breakout within just a few moves by bouncing the ball into the nook above the blocks where it can ricochet between them and the ceiling. Yet, AI has made little progress in learning how to apply general rules across games with a similar premise. A separate model has had to be trained afresh for each game in the Atari repertoire.
In contrast to AI, the brain learns quickly when thrown into new situations. It's long been understood that the brain is able to track how large a reward it anticipates receiving from a given action. If the reward is greater than expected, then the action that led to it is reinforced by the release of the neurotransmitter dopamine from neurons in the midbrain. In addition to this basic "reinforcement learning" system, the prefrontal cortex also represents actions, expectations, and rewards in the activity of its neurons. Until recently, there was no overarching model to account for how the two systems work together to enable us to learn how to learn.
What is reinforcement learning?
<<
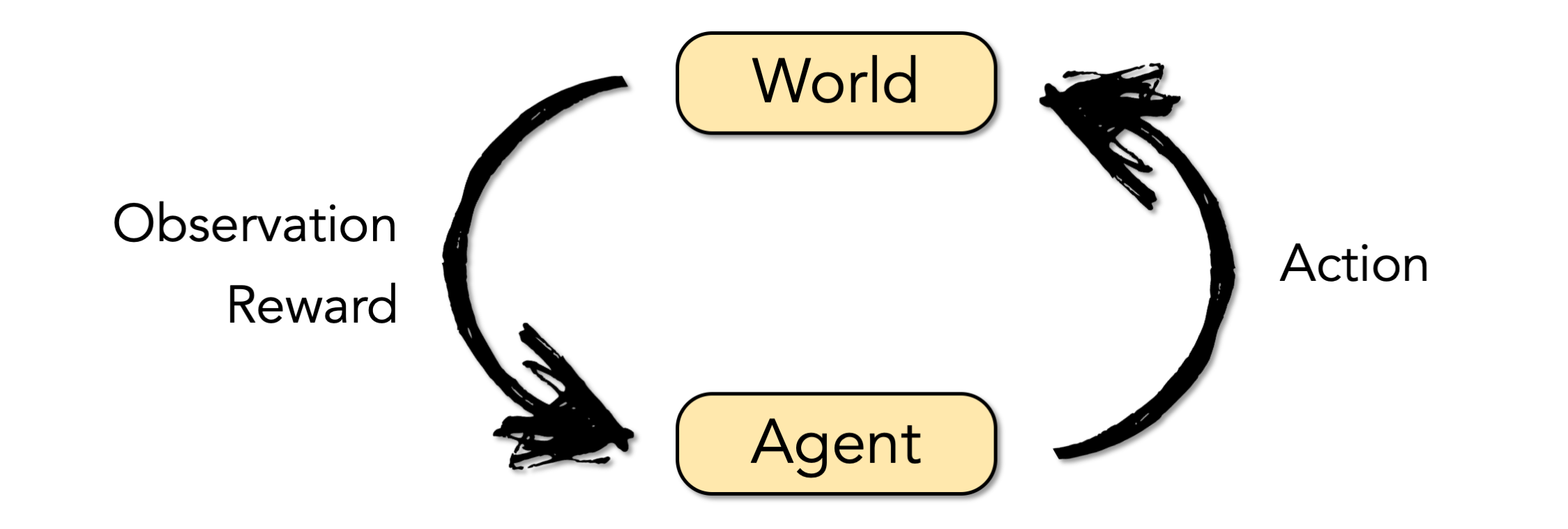
"Reinforcement learning" is a cycle of observing the world and taking actions that alter the world in order to maximize some reward. Observations are key to this cycle because they make it possible to learn which actions are most likely to yield a reward in a given set of circumstances. The agent in the reinforcement learning cycle may be a person, animal, or even a computer model.
At DeepMind, researchers leverage knowledge of how the brain learns in order to develop more efficient AI models. Their director of neuroscience research, Matt Botvinick, has likened this strategy to understanding how birds fly in order to build better planes. While there are plenty of differences between birds and planes, there are physical principles (e.g., lift) that may be gleaned from the natural implementation of flying and then applied to engineer powerful new technologies.
In a recent publication, DeepMind advanced this process of innovation one step further, showing that their new model "plane" (i.e., AI model) had achieved a property of natural "flight" (i.e., the brain's ability to learn how to learn).2 They started with the premise that the brain learns using the dopamine-based reinforcement system described above. One step further, Botvinick and his team theorized that dopamine neurons could set the synaptic weights in a second, meta-reinforcement learning system in the prefrontal cortex. They modeled this dual learning system using a recurrent neural network with simulated “dopamine” inputs.
- Ventral Tegmental Area
Contains the cell bodies of dopamine neurons in the mesocortical pathway (teal) and mesolimbic pathway (not shown). - Mesocortical Pathway
Dopamine neurons projecting from the ventral tegmental area to the prefrontal cortex. - Mesocortical Pathway
Dopamine neurons projecting from the ventral tegmental area to the prefrontal cortex. - Mesocortical Pathway
Dopamine neurons projecting from the ventral tegmental area to the prefrontal cortex. - Substantia Nigra
Contains the cell bodies of dopamine neurons in the nigrostriatal pathway. Its name translates to black substance in Latin, referring to the dark pigment released in the process of dopamine synthesis. - Nigrostriatal Pathway
Dopamine neurons projecting from the substantia nigra to the caudate and putamen, which together make up the striatum.
Midbrain dopamine neurons are classically thought to support reinforcement learning. The prefrontal cortex is thought to "learn how to learn" by implementing a meta-reinforcement learning algorithm. Its neural connections are weighted by input from dopamine neurons running in the mesocortical pathway. In addition to the role of dopamine in learning, there are dopamine neurons running in the nigrostriatal pathway which facilitate movement, and in the mesolimbic pathway (not shown) which support emotional processing.
The researchers showed that their model was able to learn like the brain by having it perform the same kinds of tasks as monkeys and humans in previous neuroscience studies. One of these tasks was adapted from a classic experiment led by Harry Harlow in 1949.3 In the original study, two objects were set before a monkey who would have to choose which one covered a food reward. The objects remained the same for six trials, over which the animal was able to learn which object was rewarded. Then, the objects were switched out for two new objects. You can play the same kind of task here:
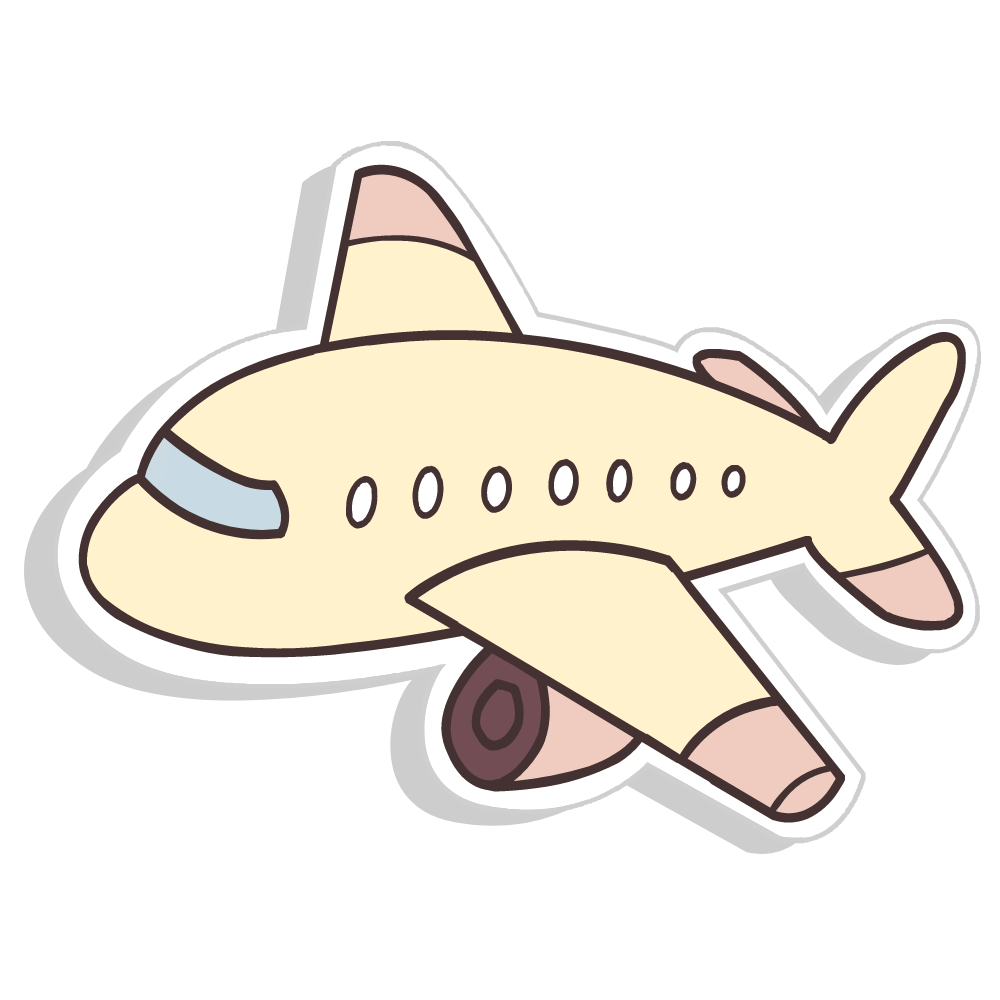
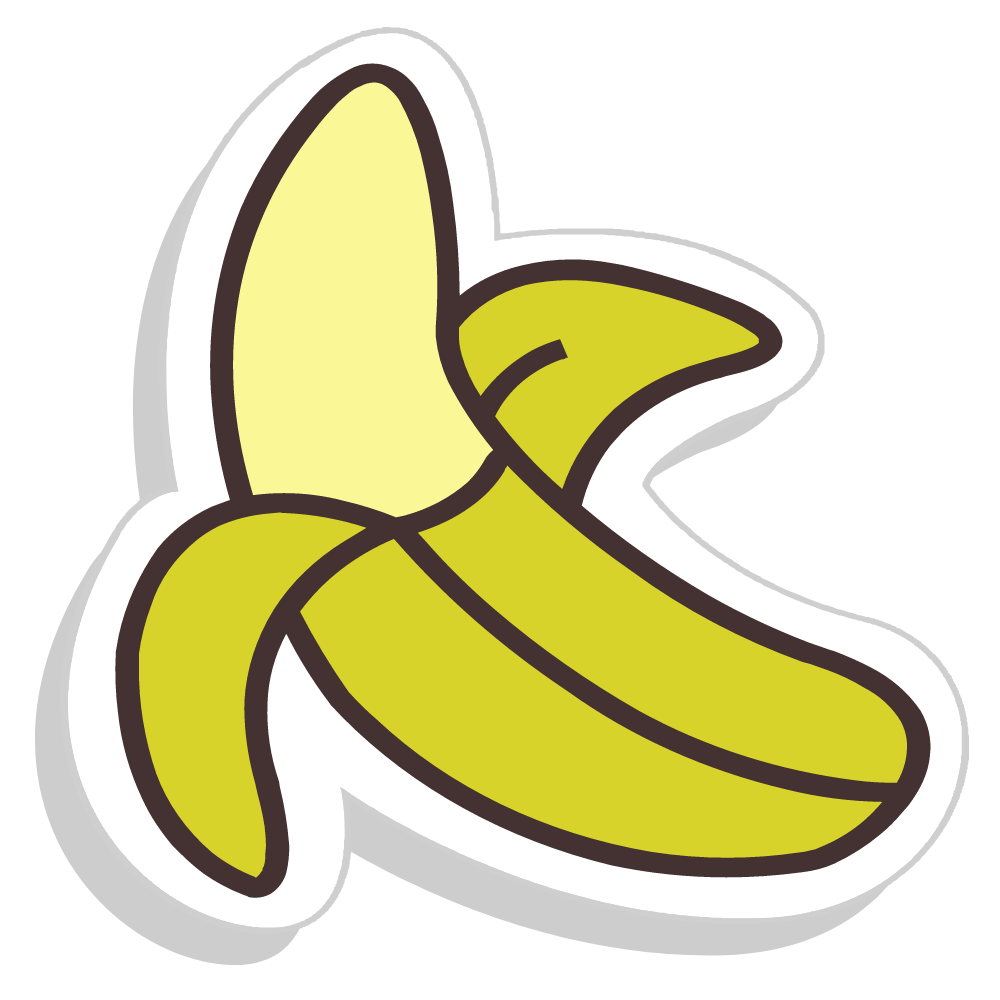
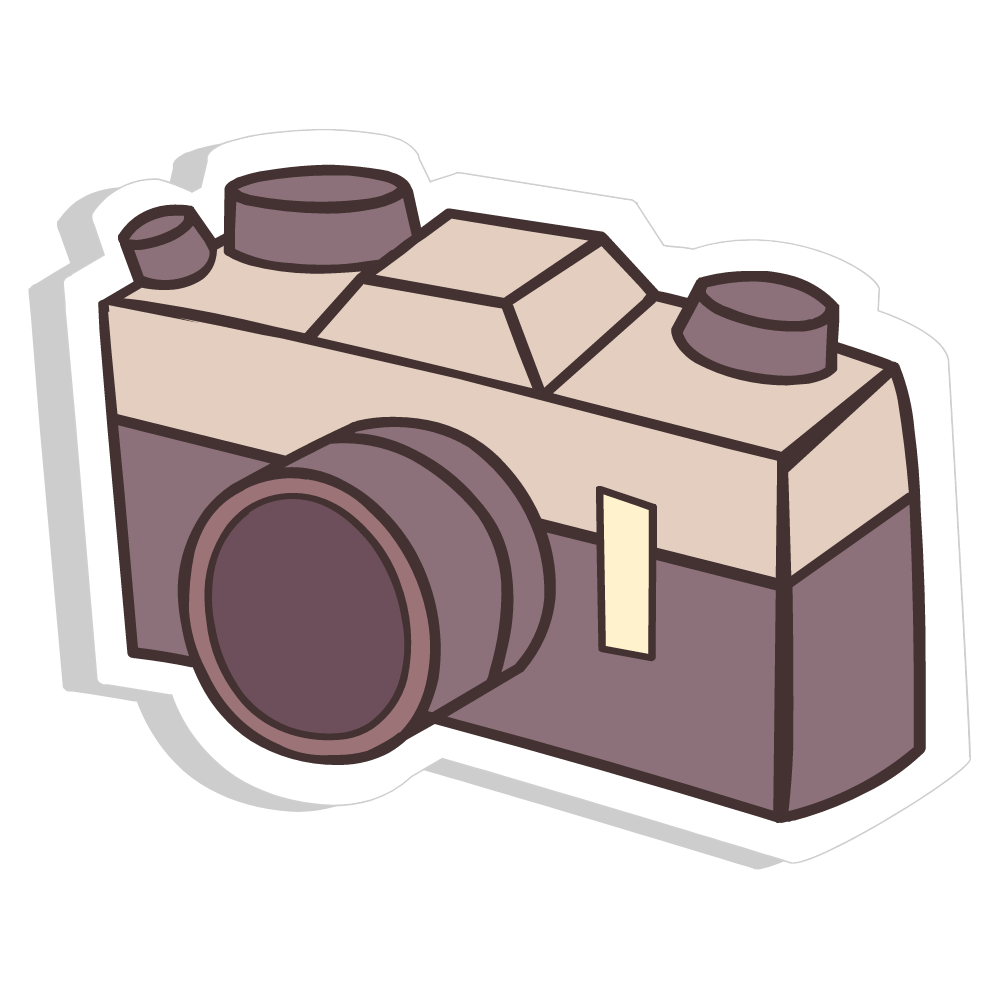
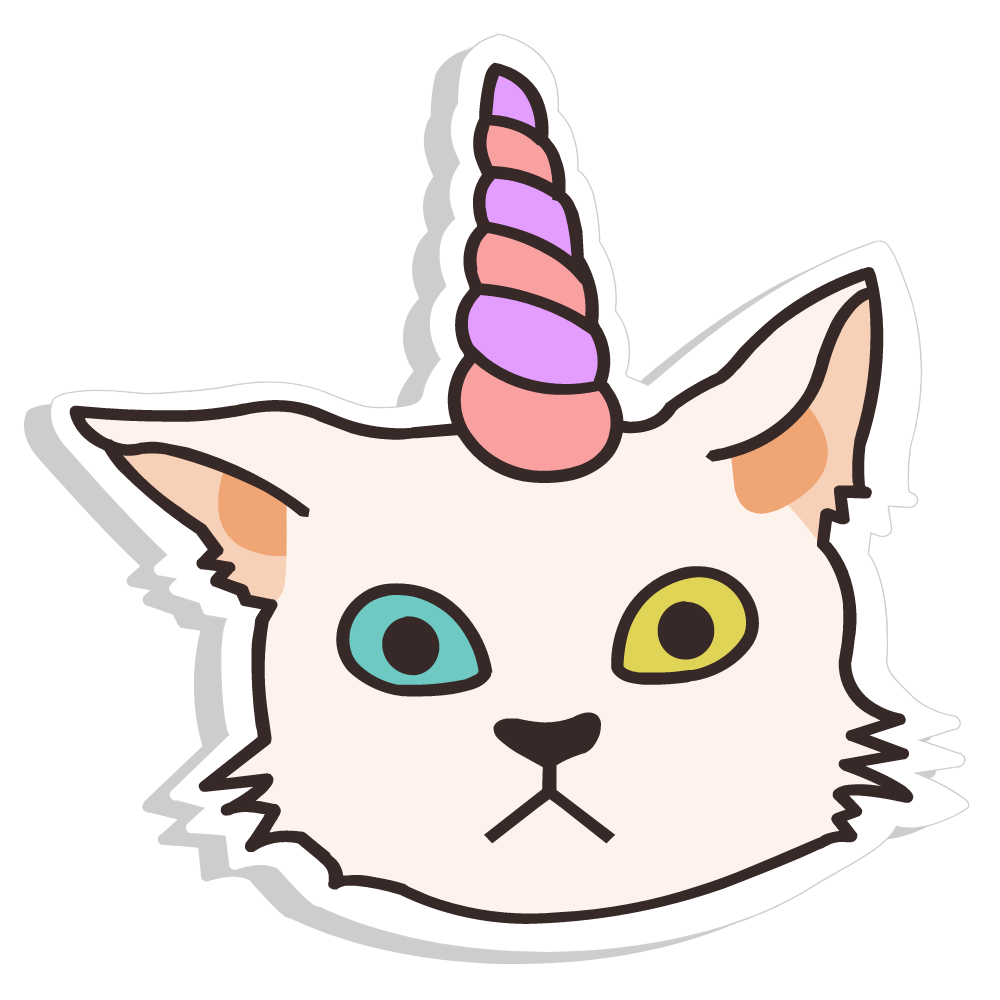
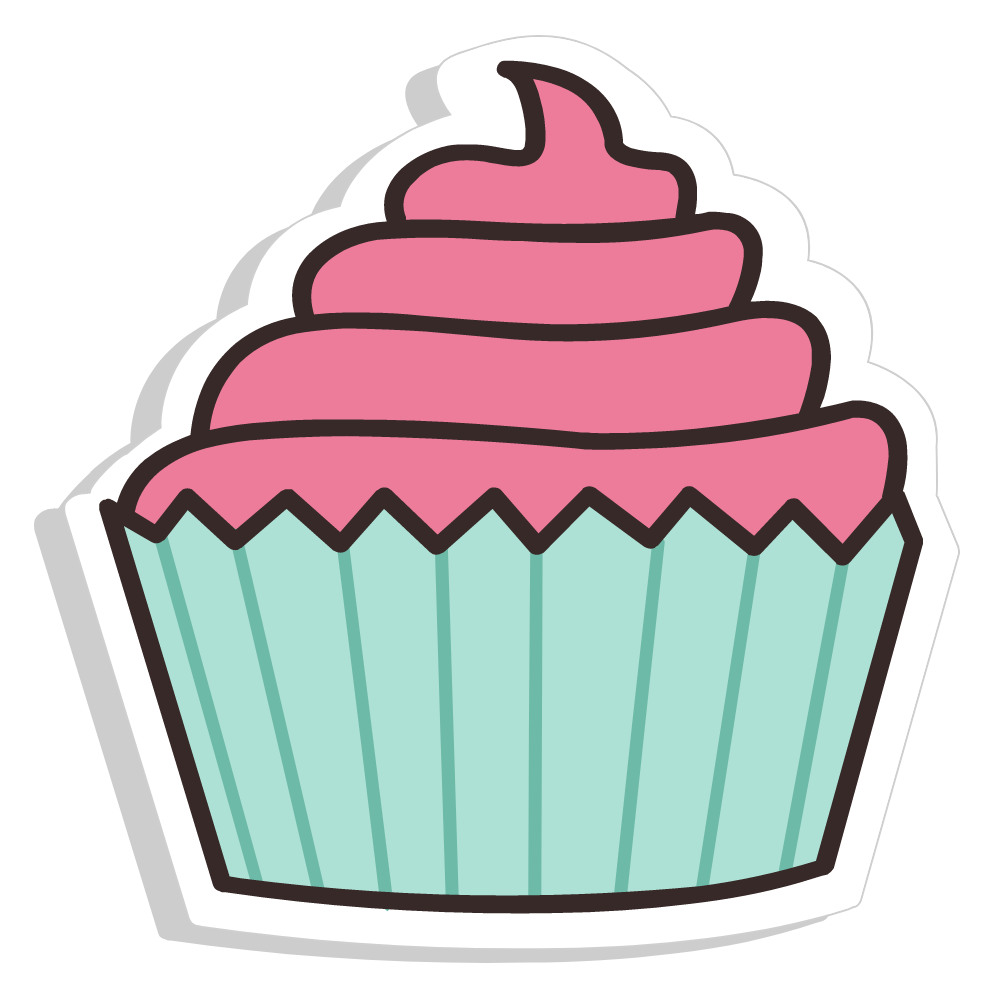
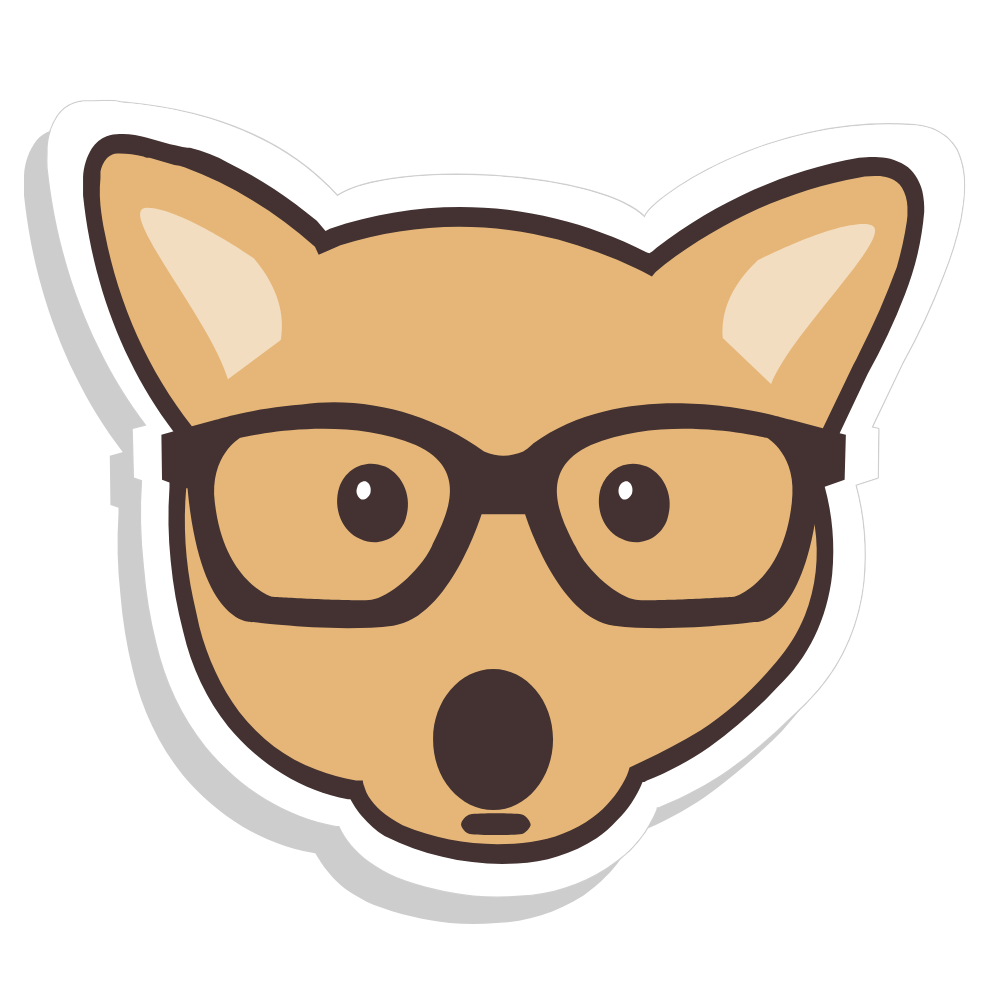
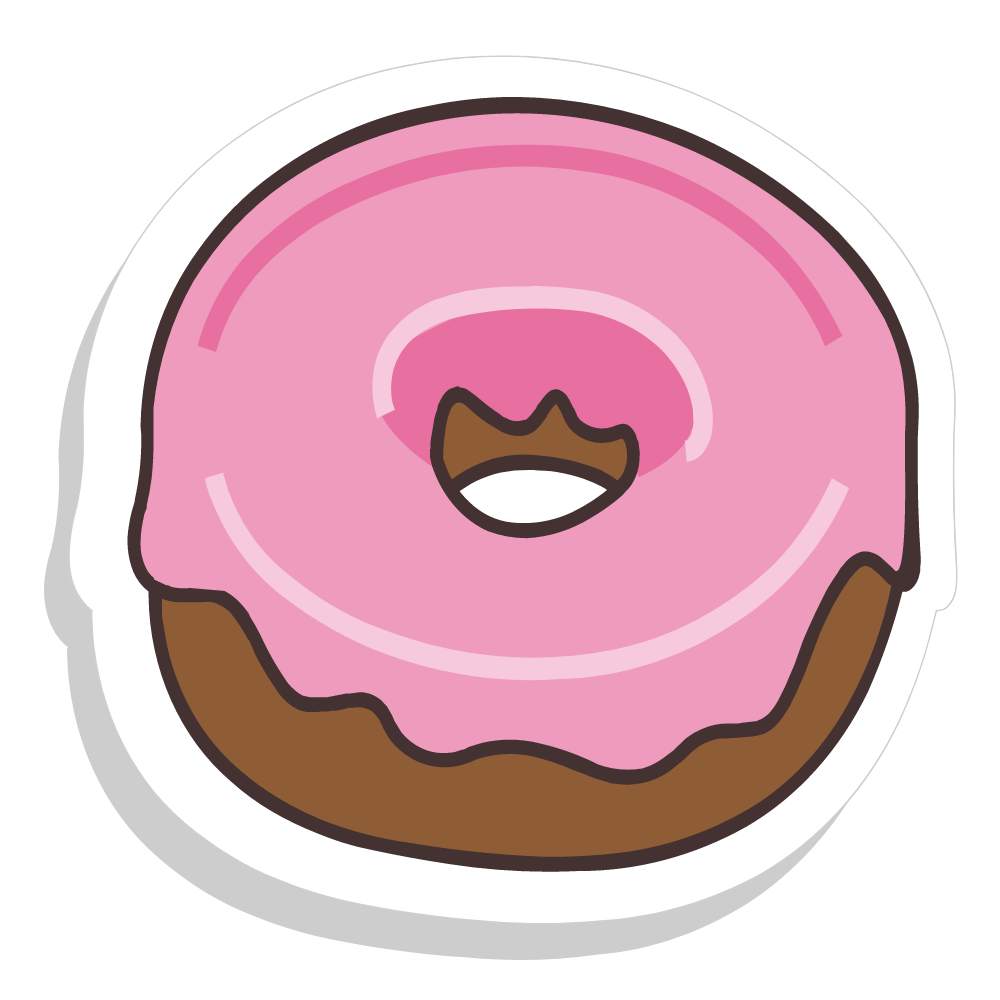
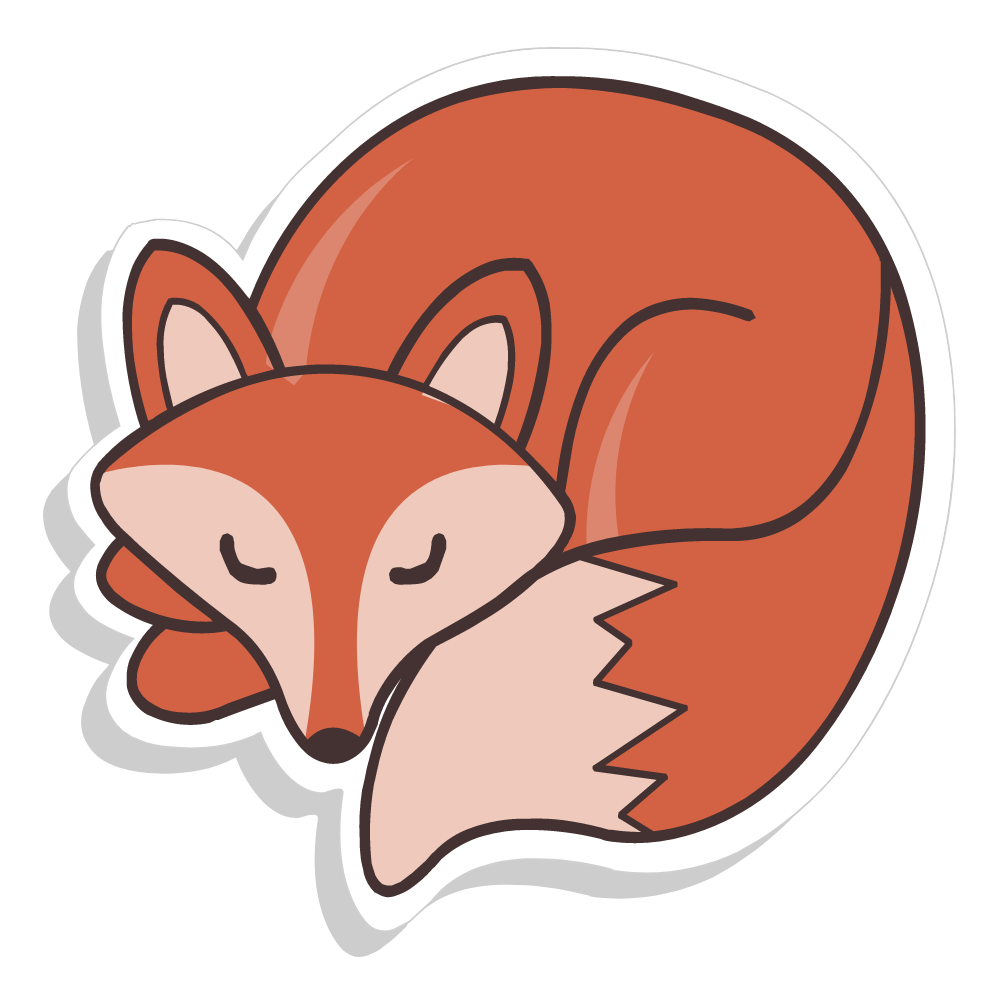
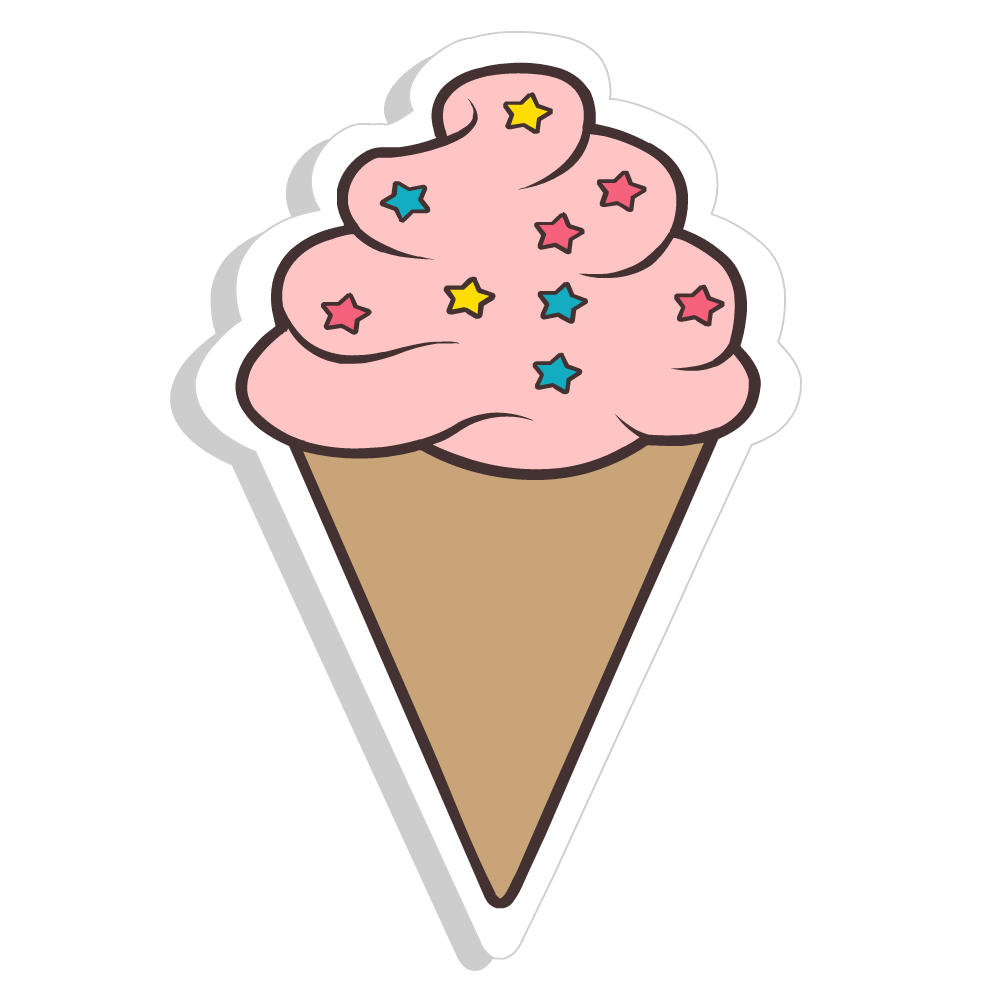
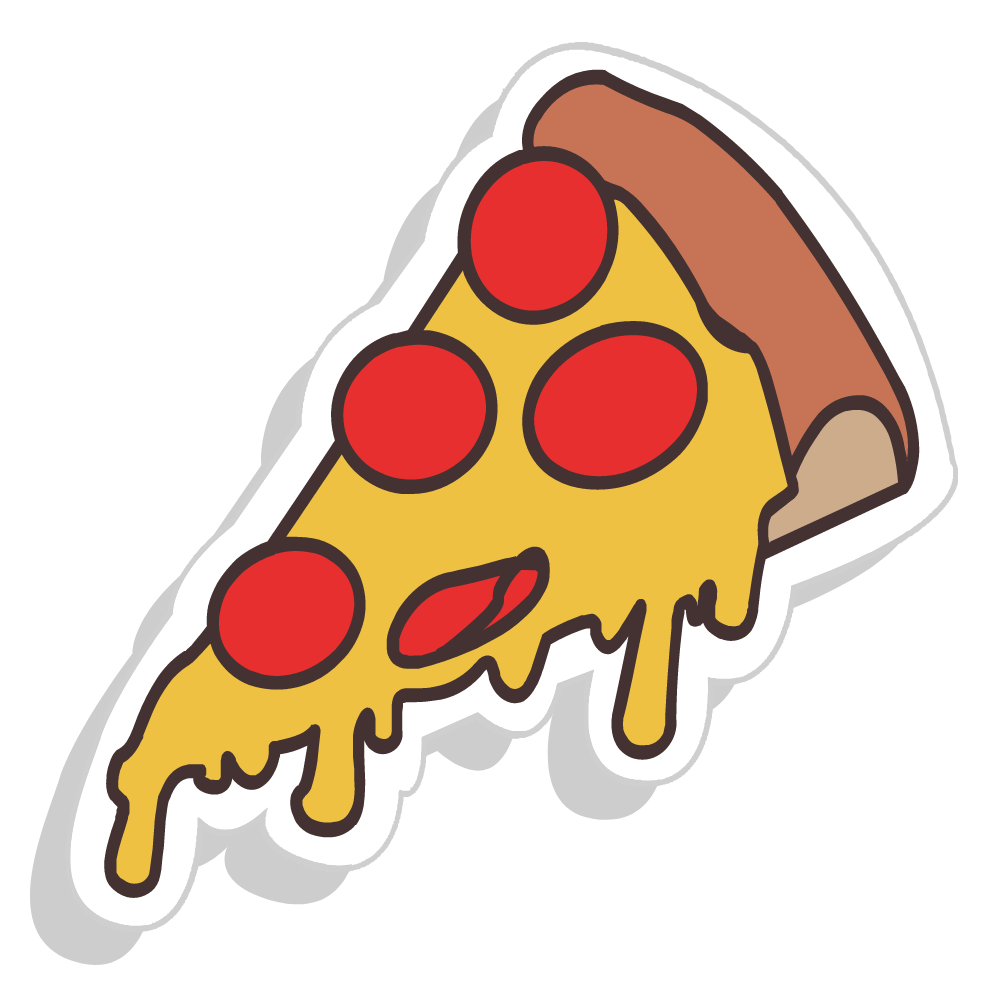
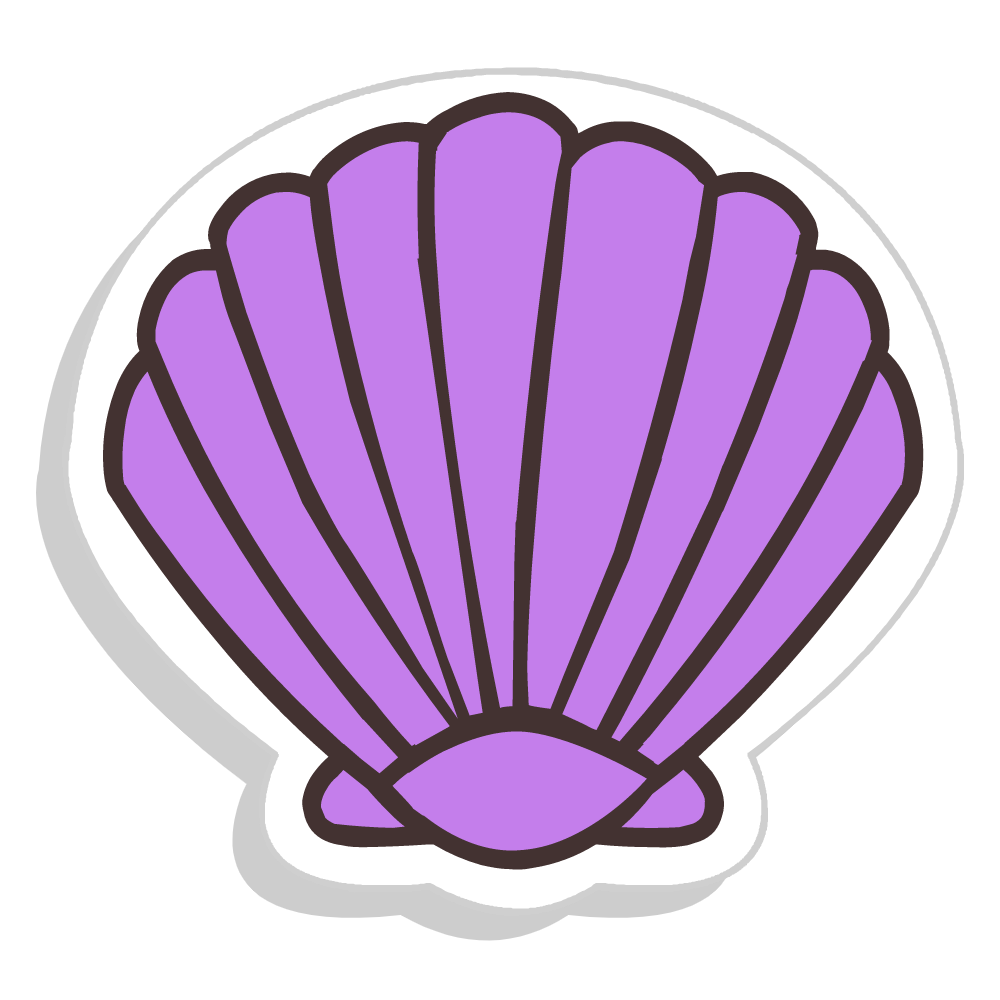
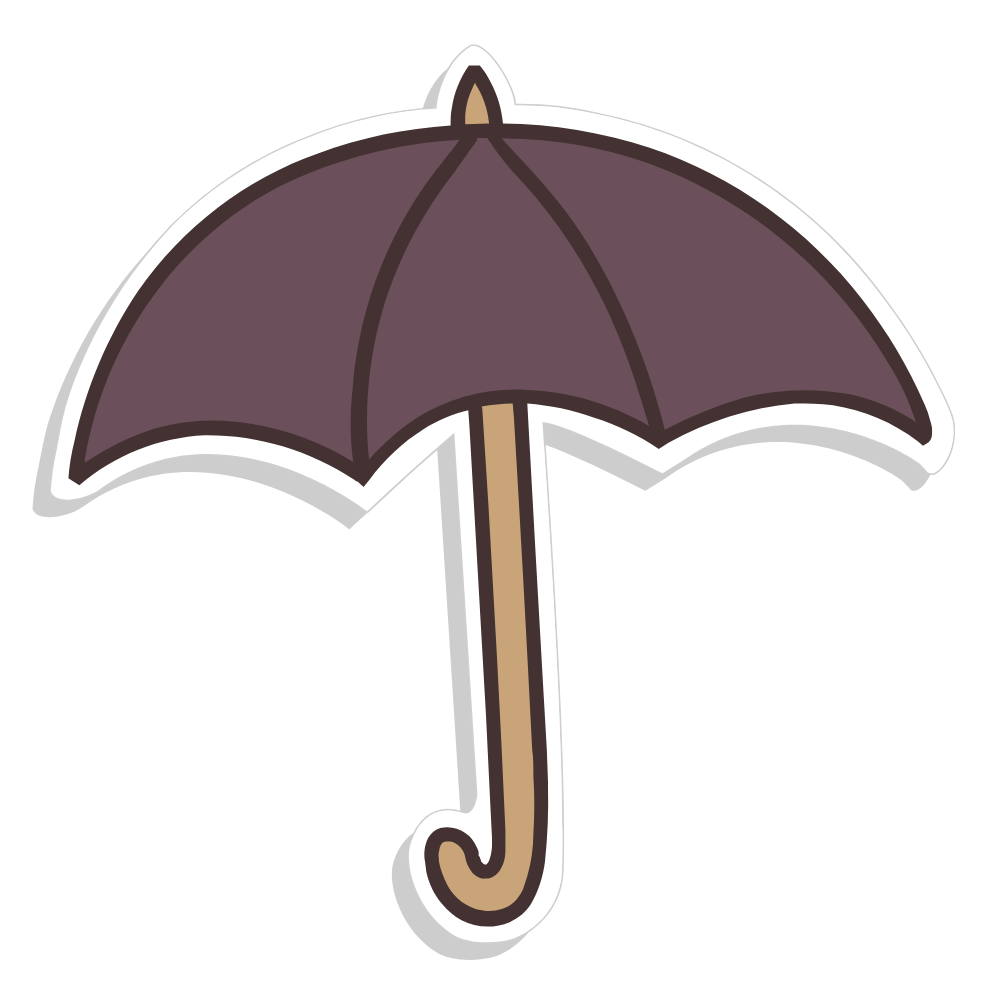
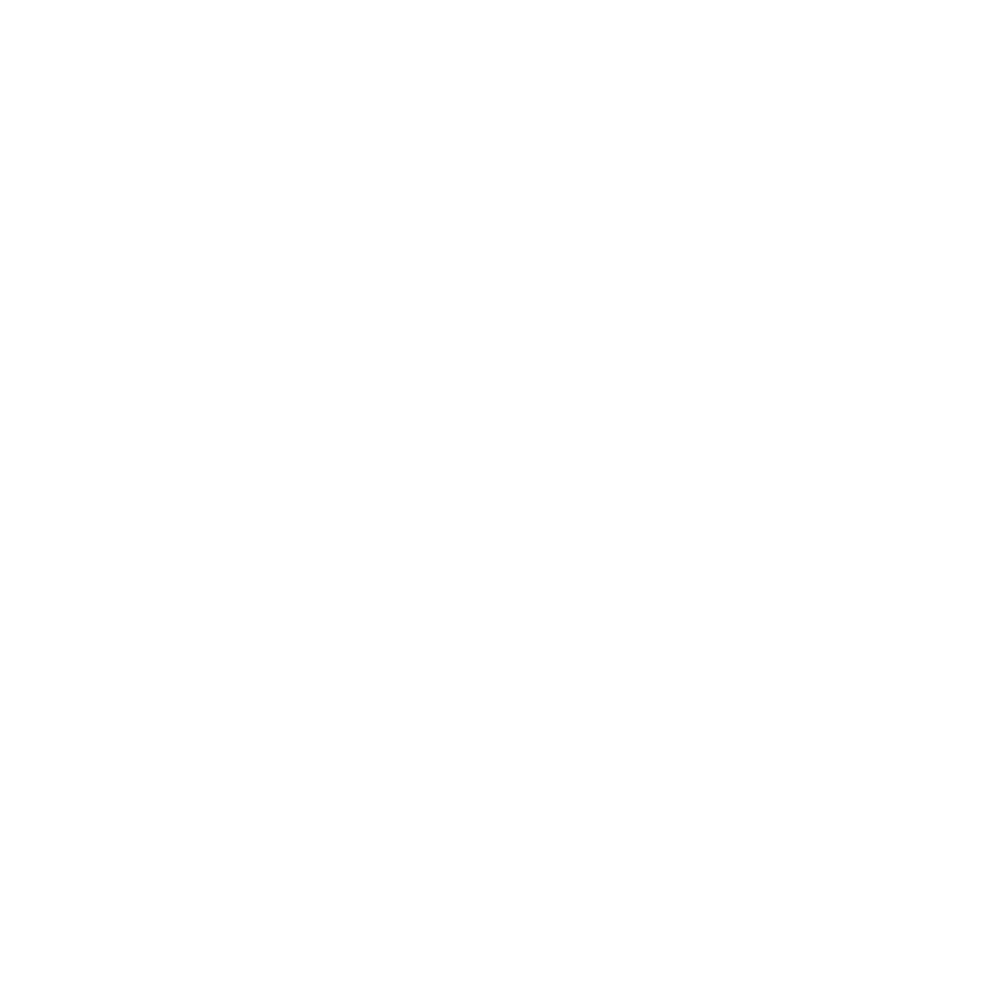
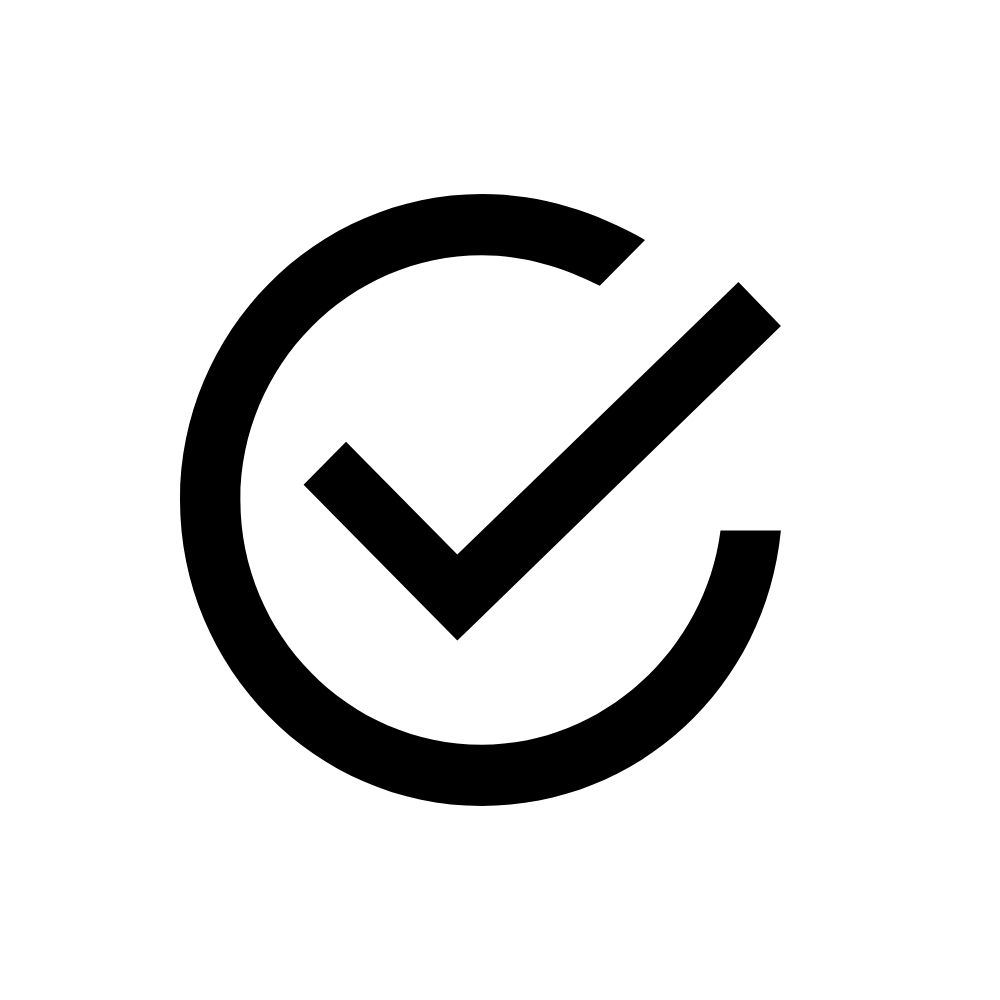
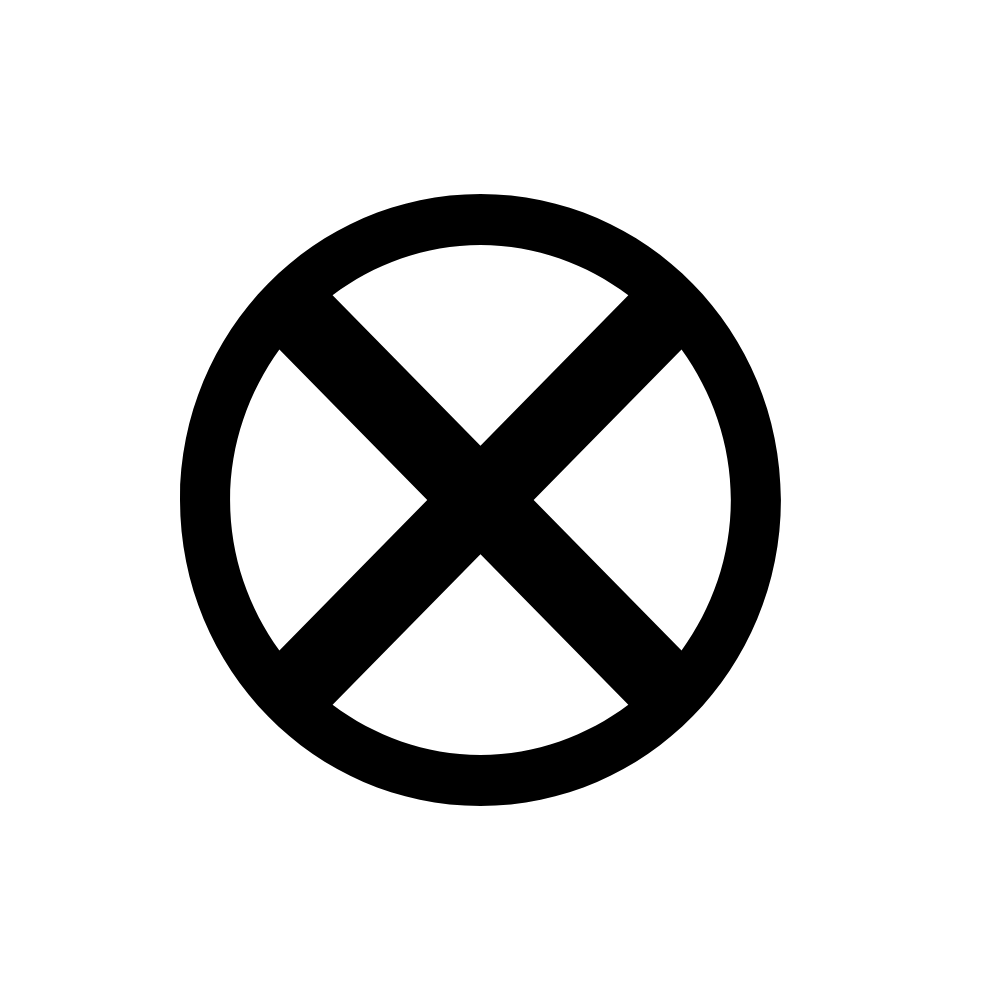
Choose an object:
Score:
Wang et al. (2018) trained a meta-reinforcement learning model to perform the same kind of task as above,1 which was originally developed by the neurophysiologist Harry Harlow in 1949.3 Over six trials, one object in each pair is rewarded. The pair of objects is then switched. In order to demonstrate "learning to learn," the model proposed by Wang et al. would have to pick up on the rule of the game that one of the two objects in each new pair would be rewarded.
When the brain performs this task, it initially chooses randomly between the objects. Over a few sets of objects, the brain is able to select the object hiding the reward after a single trial. This is an example of learning how to learn by applying an abstract rule from one scenario to another. Remarkably, the data recorded over the course of training Botvinick's meta-reinforcement learning model showed the same pattern as that recorded from behaving animals. In other words, the model could replicate the brain's process of learning how to learn.
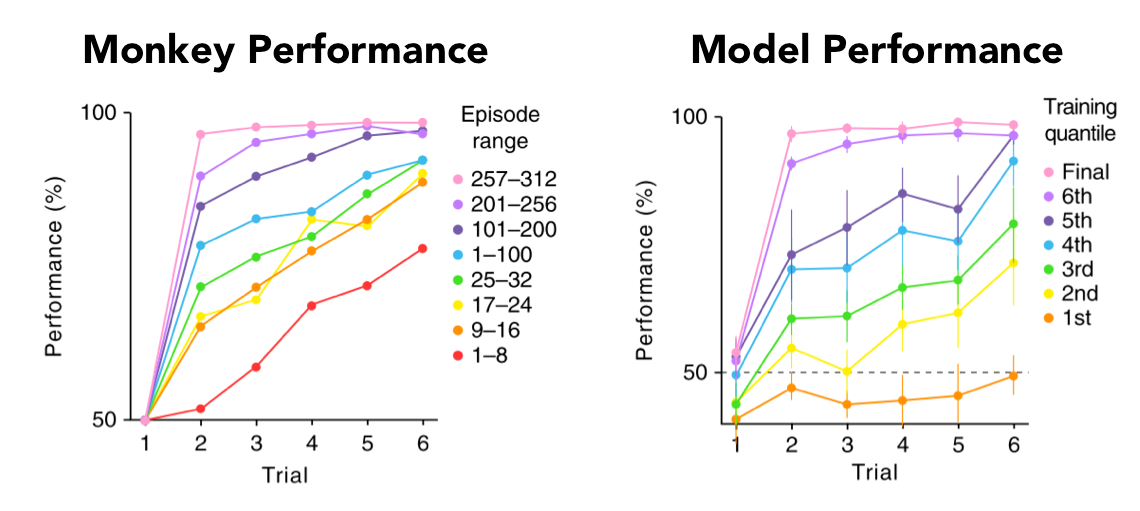
Performance on the Harlow task in terms of accuracy, computed as the percentage of objects that were correctly selected. The overall pattern for improvement in accuracy over the course of learning was similar between the monkey (left) and the computer model (right). Adapted from Figure 6 of Wang et al.2
This line of research represents a fruitful intersection of computer science and neurobiology. However, if your interest is in understanding the brain, you may feel a tad dissatisfied. The researchers did not demonstrate that the brain necessarily implements a meta-reinforcement learning algorithm in its prefrontal cortex. Future researchers will need to offer evidence that dopamine neurons send signals to the prefrontal cortex that are indispensable for learning how to learn. One way to do this would be to disrupt dopamine inputs to the prefrontal cortex and show that animals can no longer improve across sets of trials in the Harlow task.
From the engineer's perspective, the results of the Botvinick study are of immediate interest in developing improved applications of AI. In the near future, more efficient reinforcement learning algorithms may facilitate dynamic and individualized medical diagnostics or accelerate the deployment of autonomous vehicles on the road. AI isn't just playing games anymore. It will be an exciting time for engineers and ethicists alike as technology advances up to and beyond the limits of our own neural mechanisms.
References
- Mnih, V. et al. Human-level control through deep reinforcement learning. Nature Neuroscience (2015).
- Wang, J. X. et al. Prefrontal cortex as a meta-reinforcement learning system. Nature Neuroscience (2018).
- Harlow, H. The formation of learning sets. Psychological Review (1949).